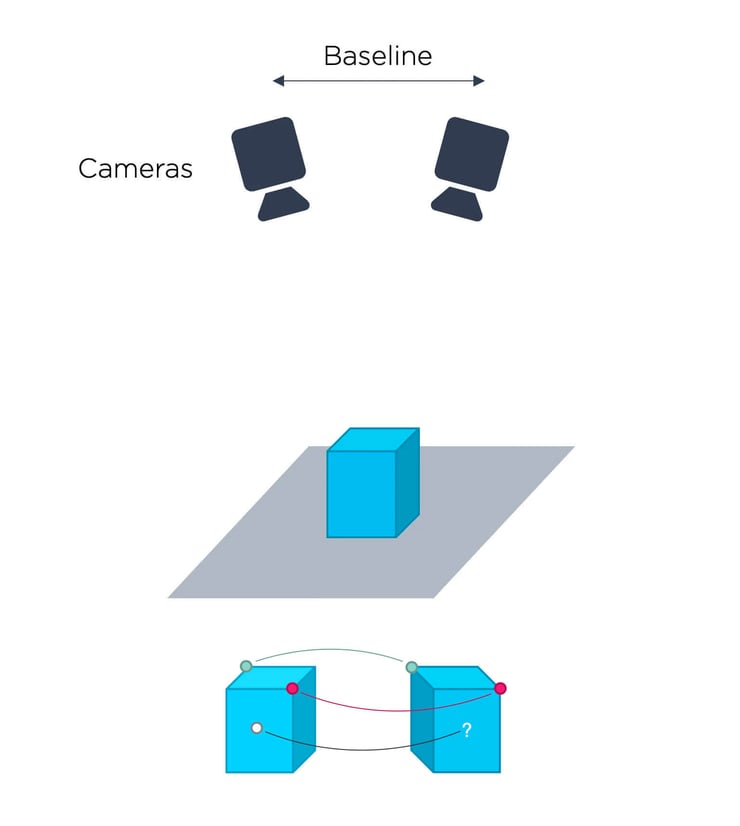
Stereo Vision
Principles
- Mimicking human vision.
- Two or more cameras.
- Triangulation. Find corresponding points in each camera.
- Disparity is proportional to depth.
Key considerations
- Passive, no active lighting required.
- Long range and outdoor usage.
- Sensitive to flat texture-less scenes.
- Less suitable for dense point cloud representations.
- Region based correspondence, block averaging.
- Look out for correspondence problem.
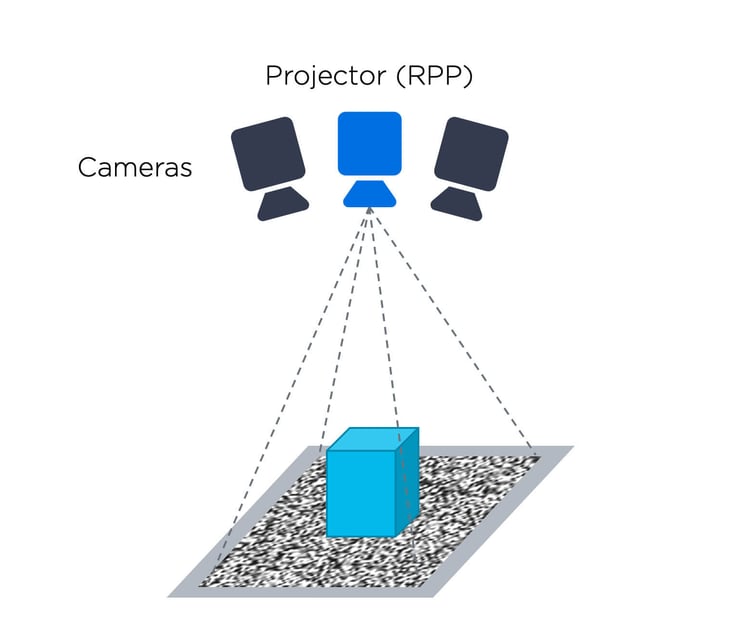
Active Stereo Vision
Principles
- Same as stereo vision.
- Additional random pattern projector (RPP).
- Mimicking human vision.
Key considerations
- Same as stereo vision, but active source.
- Shorter range
- Sensitive to ambient / sunlight.
- No texture on surfaces required.
- Solves correspondence problem.
Compare stereo and structured light vision point clouds.
Stereo- and active stereo cameras are often used by AI algorithms in bin-picking applications. Here are examples of a stereo camera point cloud and the same scene captured with a time-coded structured-light camera (like Zivid).
Keep learning!
Read the whole article about active and passive 3D vision principles here, including stereo, active stereo, laser, ToF, and structured light machine vision.